views
The journey of a cable is surprisingly complex. It starts with sourcing raw materials like copper or polymers, often from different corners of the globe. Then comes intricate manufacturing, warehousing diverse product types, and finally, distributing finished cables through various channels to customers worldwide – perhaps supporting rapid infrastructure growth in places like India. Managing this intricate dance traditionally involved spreadsheets, experience, and perhaps some gut feeling. But in today's data-rich world, there's a smarter approach: Big Data Analytics. By collecting, analyzing, and acting on vast amounts of information, cable companies can gain unprecedented insight into their operations, smoothing out kinks and driving significant improvements in efficiency.
What Exactly is "Big Data" in This Context?
It’s not just about having lots of data; it’s about dealing with data that has specific characteristics, often called the "Vs":
-
Volume: The sheer scale of data generated daily – from factory floor sensors (MES), warehouse scanners (WMS), shipping logs (TMS), enterprise planning systems (ERP), supplier portals, IoT devices tracking shipments, and even external sources like market reports or weather data.
-
Velocity: Data arriving at incredible speed, demanding near real-time processing – think live updates on truck locations or instant alerts on production line status.
-
Variety: Data coming in all shapes and sizes – structured numbers in databases (order quantities, inventory levels), semi-structured logs (sensor readings with timestamps), and unstructured information (emails from suppliers, customer feedback, news articles about port congestion).
-
Veracity: The critical need for the data to be accurate and reliable – basing decisions on flawed data leads to flawed outcomes.
The challenge and opportunity lie in effectively capturing, processing, and extracting meaningful insights from this complex data landscape.
How Analytics Translates Data into Efficiency
Applying analytical tools and techniques to this data can revolutionize supply chain performance:
1. Forecasting Demand with Higher Accuracy
-
Move beyond simple historical averages. Sophisticated algorithms can analyze past sales data alongside current market trends, economic indicators (like construction or manufacturing indices), customer-provided forecasts, known seasonality, promotional activity, and even external factors (like planned government infrastructure projects) to predict future demand far more accurately.
-
Impact: This minimizes the costly problem of holding excessive inventory for slow-moving products while reducing the risk of stockouts and lost sales for high-demand cables.
2. Optimizing Inventory Levels
-
Gain a clear, real-time, consolidated view of all inventory – raw materials, work-in-progress (WIP), and finished goods – across multiple factories, warehouses, and potentially even distribution hubs.
-
Analytics can calculate the optimal stock level for each specific cable type (SKU) based on its demand forecast, supplier lead times, production cycles, and desired customer service levels, thus minimizing capital tied up in inventory while ensuring availability.
-
Quickly identify slow-moving or potentially obsolete stock for targeted promotions or disposition strategies.
3. Improving Supplier Management
-
Systematically track and analyze key supplier performance indicators (KPIs): on-time delivery rates, incoming material quality consistency (linking QC data to specific supplier batches), lead time reliability, and pricing trends.
-
Objectively identify top-performing suppliers versus those presenting potential risks. This data strengthens negotiating positions and enables deeper, more collaborative relationships with reliable partners, such as established quality cable suppliers in uae.
4. Streamlining Logistics and Transportation
-
Analyze historical shipping data to identify the most cost-effective and reliable transportation routes and carriers, considering fuel costs, transit times, typical traffic patterns (especially crucial in congested areas), and delivery reliability records.
-
Optimize truck or container loading to maximize space utilization and reduce per-unit shipping costs.
-
Use real-time tracking data (from GPS/IoT) combined with predictive analytics (e.g., factoring in weather forecasts) to anticipate potential delays and proactively manage exceptions, improving communication with customers.
5. Proactive Risk Identification and Mitigation
-
Monitor a broad range of internal and external data sources – supplier financial health indicators, shipping lane congestion reports, weather alerts, geopolitical news feeds, commodity price trends – to detect early warning signs of potential supply chain disruptions.
-
Analytics platforms can flag emerging risks, giving supply chain managers time to activate contingency plans, such as securing alternative transport, verifying backup supplier capacity, or strategically increasing safety stock for certain items.
6. Aligning Supply with Production Needs
-
Integrate real-time supply chain visibility (e.g., expected arrival time of a critical polymer shipment) directly with factory production scheduling systems (MES).
-
Helps ensure that the right materials are available at the right time, preventing costly production line stoppages caused by material shortages and enabling smoother manufacturing operations, vital for efficient facilities like those run by leading cable manufacturers in uae.
The Technology Behind the Insights
Leveraging Big Data effectively typically involves a combination of technologies:
-
Data Warehouses or Cloud-based Data Lakes capable of storing and managing large, diverse datasets.
-
Business Intelligence (BI) tools and Analytics Platforms (ranging from specialized supply chain software to broad platforms from vendors like SAP, Oracle, Microsoft, or Tableau) for data visualization and reporting.
-
Advanced Analytics techniques, including Artificial Intelligence (AI) and Machine Learning (ML), for predictive modeling, optimization algorithms, and anomaly detection.
Embarking on the Data-Driven Path
Implementing Big Data analytics is a strategic journey:
-
Start with the Problem: Clearly define the specific business challenges you aim to address (e.g., reduce late deliveries, improve inventory turns).
-
Identify & Integrate Data: Locate the relevant data sources across different departments and systems (often a major hurdle) and work on consolidating or connecting them. Prioritize ensuring data quality.
-
Choose the Right Tools: Select analytics platforms and technologies that fit your specific needs, budget, and technical capabilities.
-
Develop Expertise: Invest in training your existing team or hire personnel with skills in data analysis, data science, and supply chain management.
-
Pilot & Iterate: Begin with smaller, focused pilot projects to demonstrate value and learn before scaling up across the entire supply chain.
-
Foster a Data Culture: Encourage and empower teams at all levels to use data to inform their decisions.
Overcoming the Hurdles
The transition isn't without challenges:
-
Breaking Down Silos: Integrating data locked away in different departmental systems (ERP, WMS, TMS, spreadsheets) can be technically complex.
-
Ensuring Data Quality: Inaccurate, incomplete, or inconsistent data ("dirty data") undermines the reliability of any analysis.
-
Security & Governance: Protecting sensitive commercial data and ensuring compliance with privacy regulations is critical.
-
Investment Costs: Acquiring technology platforms and skilled talent requires budget allocation.
-
Change Management: Shifting from traditional, experience-based decision-making to relying on data requires cultural adaptation and process changes.
Conclusion: Transforming Insight into Action
Big Data analytics offers cable manufacturers a powerful toolkit to navigate the complexities of modern supply chains. By harnessing the wealth of available data, companies can move beyond simply reacting to problems towards proactively anticipating challenges, optimizing operations, and making smarter, faster decisions. It’s about converting raw data into actionable intelligence that drives tangible improvements in efficiency, cost reduction, risk management, and ultimately, competitive advantage in a demanding global marketplace.
Your Big Data Supply Chain Questions Answered (FAQs)
-
What types of data are most valuable for analyzing a cable supply chain?
It's usually a combination. Key data includes historical sales data, customer forecasts, real-time inventory levels (raw materials, WIP, finished goods), production output data, supplier performance metrics (on-time delivery, quality rejects), logistics information (transit times, freight costs, carrier performance), and sometimes external market or weather data. -
Is Big Data analytics just about generating more complex reports?
No, it goes far beyond basic reporting. While providing better visibility (descriptive analytics) is important, the real power lies in predictive analytics (forecasting what is likely to happen, e.g., predicting demand or potential delays) and prescriptive analytics (recommending optimal actions, e.g., suggesting inventory adjustments or alternative shipping routes). -
Can smaller cable companies benefit from Big Data analytics?
Yes. While they might not implement massive, enterprise-wide systems initially, smaller companies can gain significant value by starting with focused analytics projects. Analyzing sales data more deeply to improve forecasting, tracking key supplier performance metrics, or optimizing inventory for high-value items can often be done using more accessible cloud-based tools or even advanced spreadsheet techniques. -
What's often the single biggest area of improvement from these analytics?
While it varies, significantly improving demand forecast accuracy often delivers the most widespread benefits. A better forecast leads to better decisions regarding inventory levels, production scheduling, raw material procurement, and logistics planning, cascading savings and efficiency improvements throughout the chain.
How does a company typically start with Big Data analytics in its supply chain?
A common approach is to identify a specific, high-priority pain point (e.g., consistently high expedited freight costs). Then, focus on gathering the relevant data for that specific issue, analyzing it using appropriate tools (start simple if needed), demonstrating measurable improvement, and using that success to build support for broader implementation.
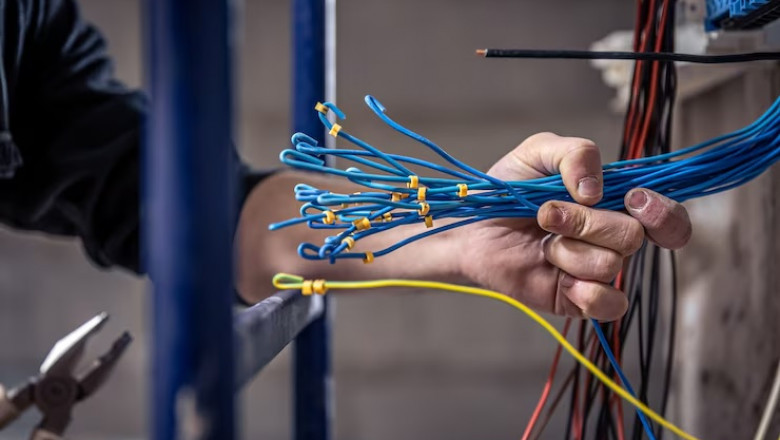

Comments
0 comment