views
Data analysts play a critical role in transforming raw data into meaningful insights that drive decision-making in businesses and organizations. Although their work often happens behind the scenes, it is essential to the success of many industries, including healthcare, finance, marketing, and technology. If you’re curious about a typical day for a data analyst, here’s a detailed look at what you can expect from this exciting career.
1. Starting the Day: Reviewing Priorities and Tasks
Like most professionals, a data analyst’s day typically begins by reviewing emails and checking in with their team or manager. Often, data analysts work as part of a larger team, which may include data scientists, engineers, or business analysts. In the morning, the first task is usually assessing the project pipeline—deciding what to prioritize based on deadlines or urgent requests.
In some cases, the analyst will also attend brief stand-up meetings (common in agile work environments), where team members discuss progress, challenges, and goals for the day. These quick meetings help the team synchronize their work and address any immediate roadblocks.
2. Data Collection and Acquisition
Once priorities are set, the first technical task for a data analyst typically involves gathering the necessary data for analysis. Data can come from a variety of sources, depending on the business and the type of analysis. Common sources include:
-
Internal databases: Data stored within the organization, often in SQL databases or cloud storage platforms.
-
External data: Data obtained from third-party services, public APIs, or purchased datasets.
-
User behavior: Website, app, or social media analytics data that help organizations understand customer interactions.
Data analysts may need to query databases using SQL, access APIs, or extract data from spreadsheets. This process often requires communicating with data engineers, who maintain the database systems and ensure that the data is clean, accessible, and structured.
3. Data Cleaning and Preparation
After collecting the raw data, the real work begins. One of the most time-consuming and challenging tasks for a data analyst is data cleaning. Raw data is often messy—containing errors, missing values, inconsistencies, or irrelevant information.
During this stage, the analyst cleans the data by:
-
Handling missing values: Filling in missing data through imputation, using averages, or deleting rows with missing values.
-
Removing duplicates: Identifying and eliminating duplicate records to ensure accuracy.
-
Standardizing formats: Ensuring consistency in date formats, numerical values, and categorical variables.
-
Dealing with outliers: Identifying extreme values and deciding whether to exclude them or investigate their cause.
Data cleaning is a critical step in the analysis process, and careful attention during this phase results in more accurate and reliable insights. A data analytics training institute in Delhi, Noida, Mumbai, and others across India can help individuals develop expertise in this area through hands-on exercises and expert guidance.
4. Exploratory Data Analysis (EDA)
Once the data is cleaned, analysts move on to Exploratory Data Analysis (EDA). This phase allows analysts to understand the data and look for patterns, trends, and insights that may inform future analysis. During this stage, the analyst uses various statistical techniques and visualizations to explore the data.
Key tasks during EDA include:
-
Descriptive statistics: Calculating means, medians, standard deviations, and other basic metrics to summarize the data.
-
Visualizations: Creating charts, graphs, and plots (using tools like Excel, Tableau, or Python’s Matplotlib and Seaborn libraries) to uncover relationships and trends in the data. For example, a scatter plot may reveal correlations between two variables, or a histogram can show the distribution of a data set.
-
Identifying patterns: Looking for patterns or anomalies that could reveal important insights or business trends.
Exploratory analysis is not always about finding immediate conclusions but rather about formulating questions and hypotheses to explore further. In some cases, analysts communicate early findings with stakeholders to refine the analysis approach.
5. Analysis and Modeling
After completing EDA, the analyst moves on to more complex tasks, such as performing statistical analyses or building predictive models. Depending on the project, this might involve:
-
Statistical analysis: Applying techniques such as regression analysis, hypothesis testing, or time series analysis to draw insights from the data. For example, an analyst might use regression analysis to understand how sales are influenced by marketing spend.
-
Building models: In some cases, the data analyst may build predictive models using machine learning algorithms, such as linear regression, decision trees, or clustering techniques. These models help predict future trends, customer behavior, or market outcomes.
-
A/B Testing: In marketing and product analysis, A/B testing is a common method used to compare two or more variations of a product, service, or website design. The data analyst evaluates the results to determine which version performed better and why.
During this stage, the analyst often works closely with other team members, such as data scientists or business stakeholders, to ensure that the model aligns with business objectives and provides actionable insights.
6. Reporting and Presenting Findings
The analysis and modeling phase culminates in creating reports or presentations that summarize key findings and actionable insights. How analysts present their results is critical because it must be understandable and relevant to non-technical stakeholders, such as managers, directors, or executives.
Analysts often use visualizations to make their findings clearer and more compelling, including charts, graphs, and dashboards that highlight key trends or outliers. Tools like Power BI, Tableau, and Excel are commonly used in this process. They may also create written reports or presentations that explain the methodology, results, and recommendations in detail.
Effective communication is a key skill for data analysts because the value of their work depends on how well their findings are conveyed and understood by others.
7. Collaboration and Feedback
After presenting the findings, data analysts often gather feedback from stakeholders, which can lead to further questions or refinements in the analysis. They may be asked to dig deeper into specific aspects of the data or conduct additional analyses.
This stage of the day often involves collaborating with cross-functional teams, such as marketing teams, product managers, or IT departments, to address any further questions and ensure that the data aligns with the business’s needs.
8. Ending the Day: Documentation and Planning for Tomorrow
At the end of the day, the data analyst typically documents their work, including any code written, analyses performed, and decisions made. This documentation is essential for future reference and collaboration with other team members.
Additionally, the analyst may spend time preparing for the next day, which could involve setting up new projects, scheduling meetings, or reviewing new data for analysis.
In The End
A day in the life of a data analyst is dynamic, involving a mixture of technical tasks, problem-solving, and communication with various teams. Data analysts play a crucial role in helping organizations make data-driven decisions. While the work can be challenging and often requires hours spent cleaning and organizing data, it is also highly rewarding, with analysts providing valuable insights that drive strategic outcomes.
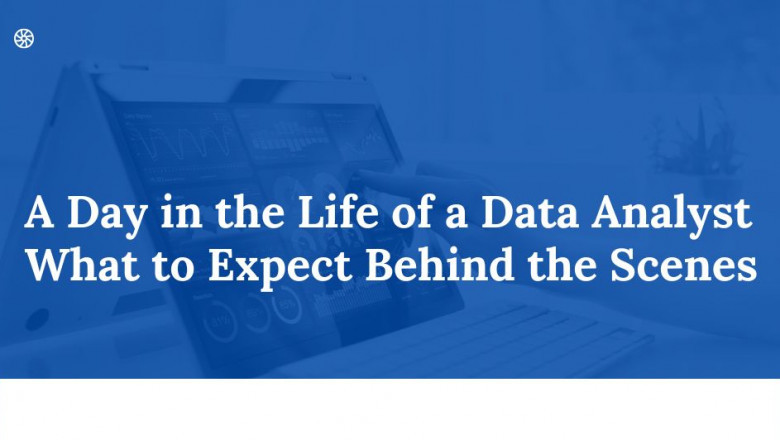

Comments
0 comment