views
Time-series analysis is a technique for data analysis aiming at extracting pertinent statistical information and traits. Predicting future value is among the key objectives of the research. Extrapolation is necessary in highly complicated forecasting with time series analysis. Still, the expected value and the related estimate of uncertainty might make the outcome quite beneficial. Join a machine learning course in Noida to gain the practical knowledge of time series analysis.
What Is Time Series Analysis?
Time-series analysis is the technique of examining a set of data points across an interval of time. Time series analysts log data points at regular intervals over a given length of time rather than sporadically or randomly.
While time-series data is information acquired over time, several kinds of information explain when and how that information was acquired. Like:
Time series data is a body of observations on the values a variable exhibits at different times.
Cross-sectional data are information from one or more concurrently gathered variables.
Cross-sectional and time-series data combined make pooled data.
Components of Time Series Analysis
Trend:
Trend shows the data's long-term directionality or movement across time. It reflects the general direction of the series—that of either increasing, declining, or stability. Trends can be nonlinear, displaying more complicated patterns, or linear, meaning a constant rise or drop.
Seasonality:
In the context of the time series, seasonality is the periodic variations or patterns occurring under consistent intervals. Usually repeating yearly, quarterly, monthly, or weekly, these cycles are impacted by seasons, holidays, or business cycles.
Cyclic Variations:
Unlike seasonality, cyclical fluctuations in the time series are longer-term variations devoid of a defined period. Usually connected with expansions and contractions in economic activity, these swings reflect business cycles, which can span several years.
Irregularity:
Irregularity, sometimes referred to as noise or randomness, is the erratic or random fluctuations in the data not ascribed to the trend, seasonality, or cyclical variations. These variances could come from additional unanticipated variables, measurement mistakes, or random events. Identification and modeling of the fundamental patterns in the time series data become difficult in irregularity.
A machine learning online course helps you in gaining the advanced skills to do time series analysis. Join the course and enhance your skills.
Time Series Data Preprocessing
Time series preprocessing is the process of cleansing, transforming, and preparing time series data for use in analysis or forecasts. Techniques meant to enhance data quality, eliminate noise, handle missing values, and render the data fit for modeling are included here. Preprocessing chores could include detrending, deseasonalizing, removing outliers, addressing missing values by imputation, data scaling or normalizing, and variance stabilization treatments used. The aim is to guarantee that the data of the time series is in a fit form for the next modeling or analysis.
Dealing with missing values in the time series data helps to guarantee continuity and dependability in analysis.
Dealing with outliers for spotting and correcting observations that greatly differ from the rest of the data, so skewing the findings of analysis.
Ensuring that the statistical features of the time series—such as mean and variance—remain consistent over time helps to guarantee stationarity and transformation. Stationarity is reached via methods like deseasonalizing, detrending, and differencing.
Benefits of Time Series Analysis
Forecast Future Patterns:
Future trend prediction made possible by time series analysis helps companies to forecast market demand, stock prices, and other important variables, therefore enabling proactive decision-making.
Detect Patterns and Anomalies:
Time series analysis helps identify repeating patterns and anomalies by looking at successive data points, therefore revealing underlying behaviors and possible outliers.
Edge of Competence:
Time series analysis helps companies—in terms of goods, personnel, or financial assets—to properly allocate resources. Businesses that keep ahead of industry trends, react to changes, and make data-driven decisions build a competitive edge.
Strategic Planning:
Time series insights enable long-term strategic planning and direct decision-making in the financial, healthcare, and other industries.
Reducing Risk:
Spotting possible hazards helps companies create plans to reduce them, thereby improving the general risk management.
Whether your area of expertise is software development or data science, time-dependent data has great value waiting to be unlocked. To learn more about it join a machine learning course in Delhi.
Conclusion
Time series analysis is a flexible instrument allowing us to find trends, grasp seasonality, project future values, and identify anomalies. From data visualization to using advanced models like ARIMA and Prophet, the trip through time series analysis is as gratifying as it is instructive.
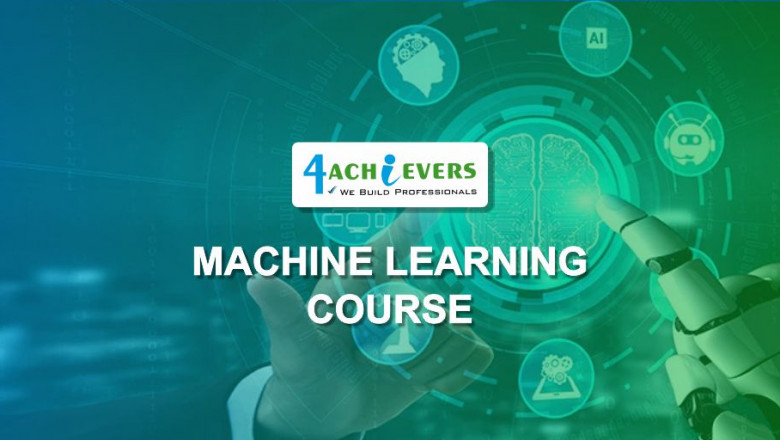

Comments
0 comment